The significance of robust fraud detection mechanisms in organizations cannot be overstated. Companies at the forefront, such as AWS Fraud Detection, have embraced cutting-edge technologies to fortify their defenses against fraudulent activities. This blog delves into the intricacies of Amazon's approach to fraud detection, unraveling the layers of sophistication employed by the tech giant. Moreover, we will shed light on the pioneering perspective brought forth by Nected, emphasizing its rules-based methodology as a transformative solution in the realm of real time fraud detection architecture.
As we navigate through this guide, the questions that are going to be answered in this blog are : What is AWS Detective? & Which AWS service can create complex graphs for fraud detection architecture? etc. We'll begin by dissecting Amazon's intricate approach to amazon fraud detection, unveiling the methodologies and technologies at play. Following this, we'll pivot towards a critical analysis, identifying the challenges and limitations that accompany the tech giant's approach.
You will then get to know about Nected, introducing its innovative rules-based methodology as a viable solution to overcome the identified limitations. The blog aims to provide a balanced understanding of both Amazon's strategies and the alternative solution offered by Nected, empowering readers to make informed decisions in the domain of of amazon fraud detection.
Amazon's Approach to Fraud Detection
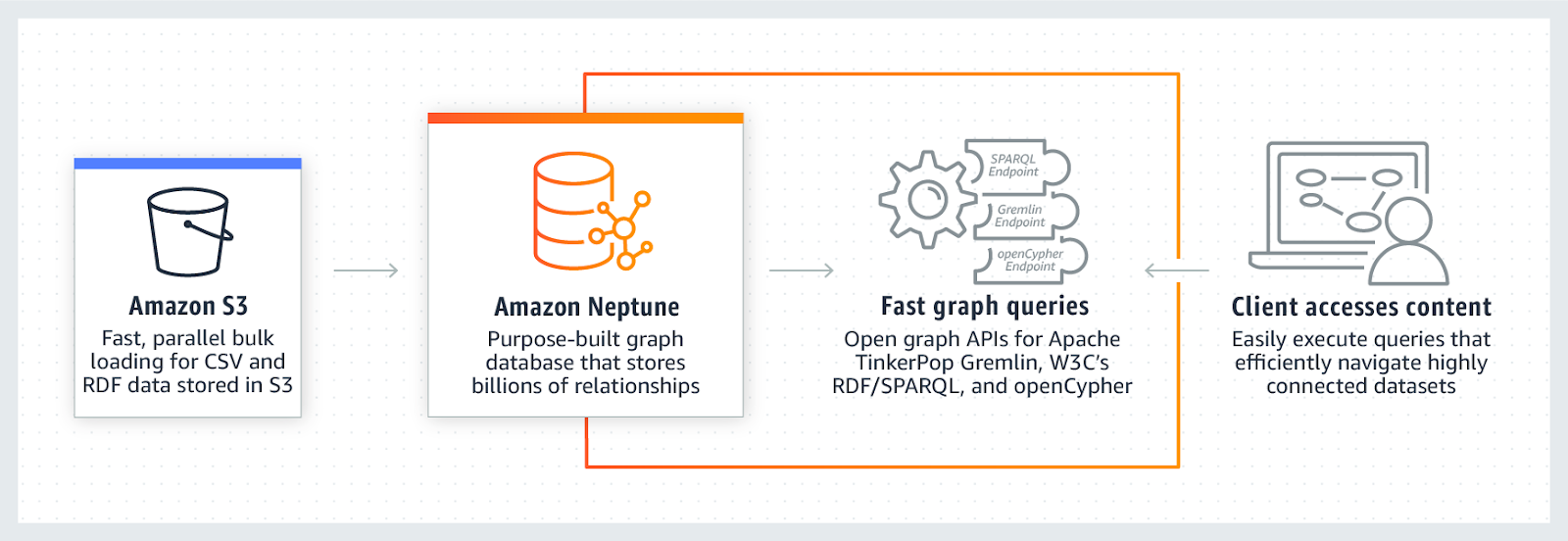
Fraud detection is a critical aspect of maintaining the integrity and security of digital platforms, and Amazon, as a tech giant, employs a sophisticated strategy to combat fraudulent activities. In this section, we will dissect the key elements of Amazon's approach, exploring the significance of graphs with Amazon Neptune, introducing AWS Detective, understanding the architectural framework of AWS Fraud Detection, and delving into the intricacies of anomaly detection.
The Significance of Graphs

Graph technology plays a pivotal role in Amazon's fraud detection framework. Traditional tabular data often falls short in representing the complex relationships that exist within datasets. Graphs, with their interconnected nodes and edges, provide a more nuanced and accurate representation of relationships between various entities. In the context of fraud detection, this allows Amazon Neptune to identify intricate patterns and anomalies that might be challenging to discern using conventional data structures.
For instance, in cases of coordinated fraudulent activities involving multiple entities, graph-based analysis becomes instrumental. It enables Amazon to uncover hidden connections, such as shared addresses, phone numbers, or other relevant information, which may not be apparent in a traditional tabular representation.
Exploring AWS Detective
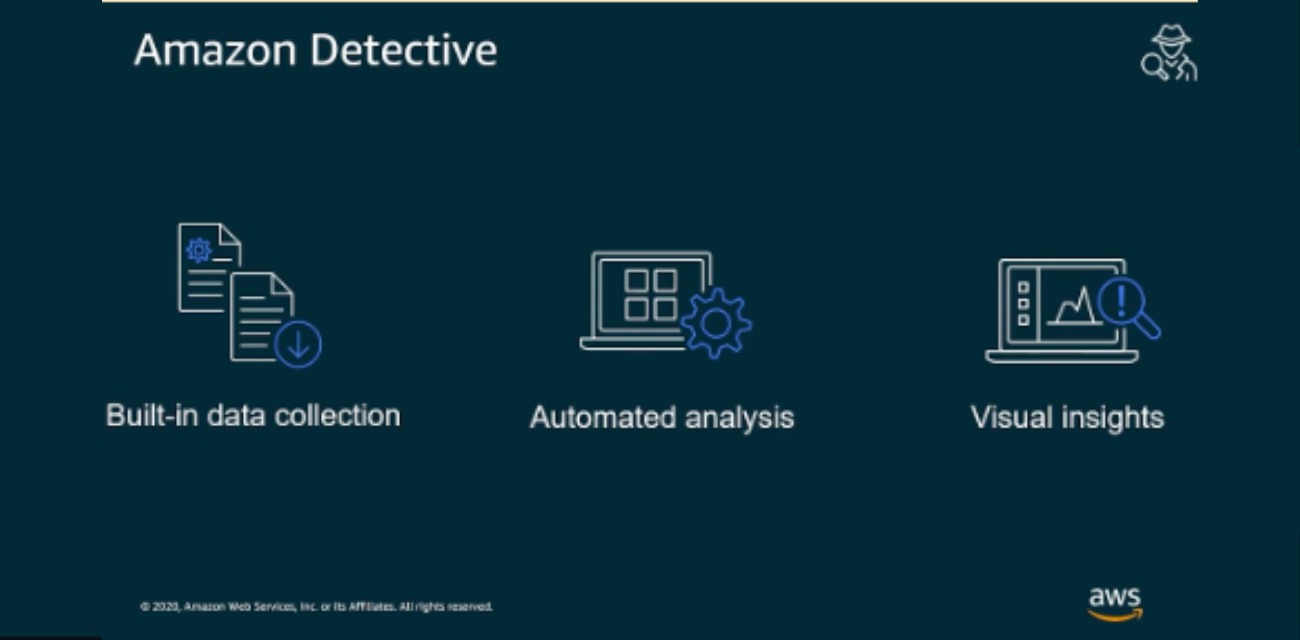
Within Amazon Web Services (AWS), the introduction of AWS Detective marks a significant leap forward in fraud detection capabilities. AWS Detective is a comprehensive security service designed to aid users in investigating and mitigating potential security issues across their AWS resources. The service utilizes advanced technologies, including machine learning and graph theory, aligning with Amazon's commitment to staying at the forefront of cutting-edge solutions for robust fraud prevention.
AWS Detective essentially acts as a force multiplier for security teams, providing a centralized platform to analyze, visualize, and respond to potential security threats. By incorporating graph theory, AWS Detective enhances the understanding of relationships between different AWS resources, allowing for a more holistic approach to security monitoring.
Architectural Insights into AWS Fraud Detection
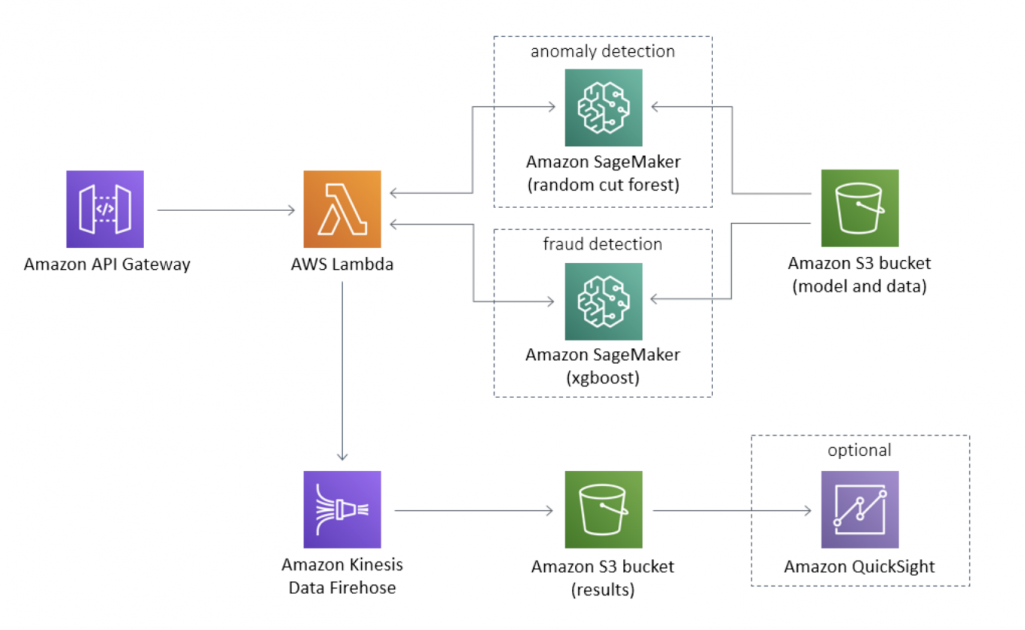
The architecture of AWS Fraud Detection involves a meticulous process guided by the data models explorer integrated into the AWS Fraud Detector console. This explorer serves as a tool to recommend suitable model types based on specific business use cases. Users navigate through this process to create and train fraud detection models, with the explorer offering insights into mandatory, recommended, and optional data elements required for an effective dataset.
Consider a scenario where the business use case is focused on new account registrations. The data models explorer recommends a specific model type for creating a fraud detection model for this use case. The model type defines the algorithms, enrichments, and transformations that AWS Fraud Detector will employ during the training phase.
Anomaly Detection in AWS
Anomaly detection stands out as a key component of Amazon's fraud detection strategy to identify potential fraudulent activities. Through the utilization of sophisticated algorithms, AWS Fraud Detector constantly analyzes patterns within datasets. The emphasis is on identifying deviations from established norms, signaling potential anomalies that may indicate fraudulent behavior.
This proactive approach allows for timely intervention, enabling Amazon to mitigate potential risks and safeguard against financial losses. Anomaly detection is particularly crucial in scenarios where fraudulent patterns might evolve over time or where traditional rule-based systems might fall short in capturing subtle variations. By leveraging advanced real-time fraud detection architecture, Amazon strives to stay ahead of evolving threats
As we navigate through these components of Amazon's fraud detection approach, it becomes evident that the tech giant employs a combination of advanced technologies to stay ahead of evolving threats. However, inherent challenges persist, paving the way for an exploration of Nected's innovative approach in the subsequent sections.
In the next section, you will uncover the limitations and challenges in Amazon's approach to fraud detection, setting the stage for Nected's rules-based methodology as a potential solution.
Read Also:- Decision Support System Examples: Improving Business Decision-Making
Challenges and Limitations
Fraud detection, despite advancements, encounters various challenges and limitations within Amazon's existing framework. In this section, you will explore the complexities associated with graph creation, shed light on the nuances of AWS Detective, highlight architectural limitations, and discuss hurdles in anomaly detection.
Complexity in Graph Creation
Creating an intricate fraud graph in Amazon Neptune involves navigating through a myriad of relationships and entities. The complexity arises from the sheer volume of interconnected data points, making it challenging to efficiently represent and navigate the intricate web of relationships. The need for a more intuitive and streamlined approach to graph creation becomes evident.
Understanding AWS Detective
While AWS Detective offers a powerful platform for security analysis, the learning curve associated with understanding its full capabilities can pose a limitation. Security teams may find it challenging to leverage the complete suite of AWS Detective's features effectively. Simplifying the understanding and implementation of AWS Detective is crucial for maximizing its potential in real-time fraud detection architecture.
Architectural Limitations
The architectural framework of Amazon Fraud Detection, while robust, is not without its limitations. The predefined data models and their recommended elements may not comprehensively cover all use cases. This can result in gaps in the fraud detection process, especially when dealing with specialized scenarios that demand a more tailored approach.
Anomaly Detection Hurdles
Anomaly detection, a pivotal aspect of fraud prevention, faces hurdles in capturing subtle variations and emerging patterns effectively. Traditional anomaly detection systems may struggle to adapt to evolving fraudulent behaviors, leading to false negatives or delayed detection. Addressing these hurdles is essential for enhancing the overall efficacy of fraud detection mechanisms.
Read Also:- Utilizing Fraud Detection Models in Modern Businesses
Nected: A Rules-Based Revolution
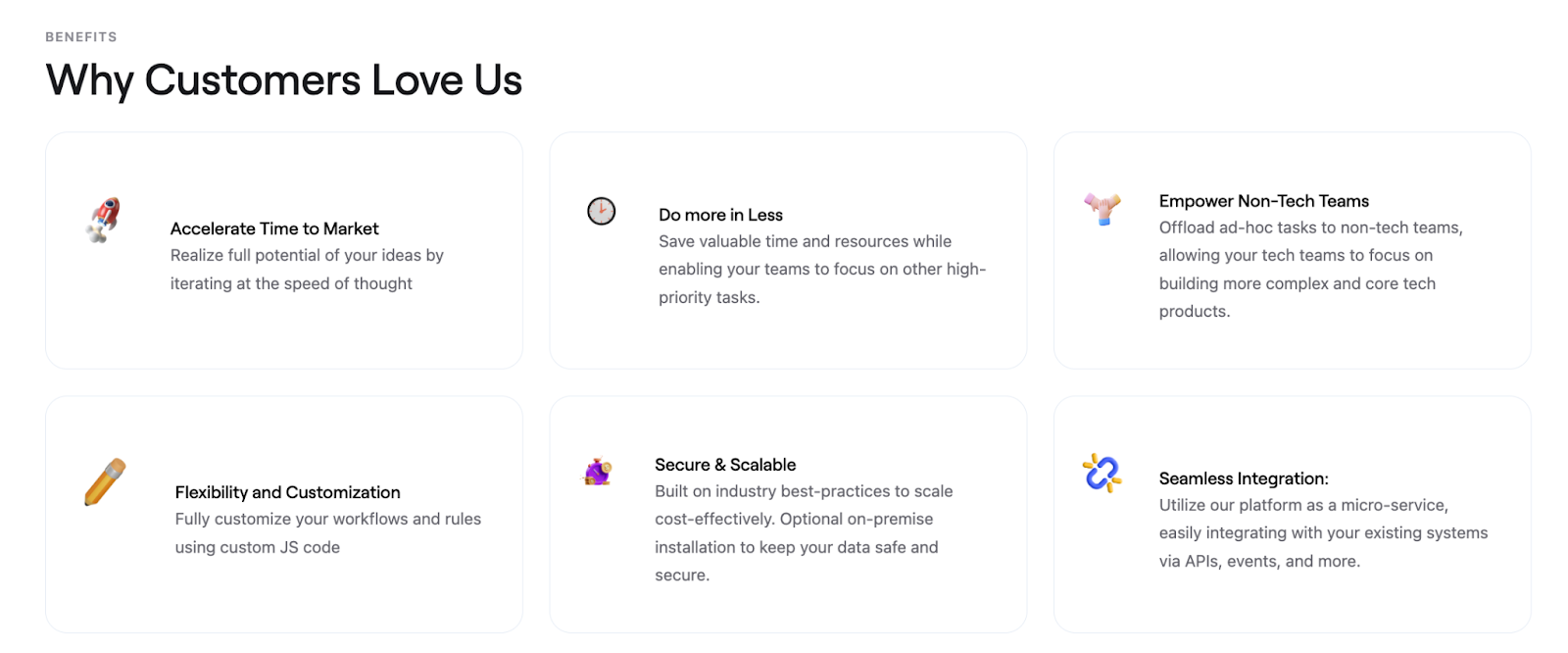
In response to the challenges posed by Amazon's approach, Nected emerges as a rules-based revolution in fraud detection. This section will unveil Nected, explore how it addresses the limitations inherent in Amazon's system, delve into the streamlined architecture of Nected, and highlight the integration of machine learning for enhanced fraud detection.
Nected's Rules based approach to Fraud Detection
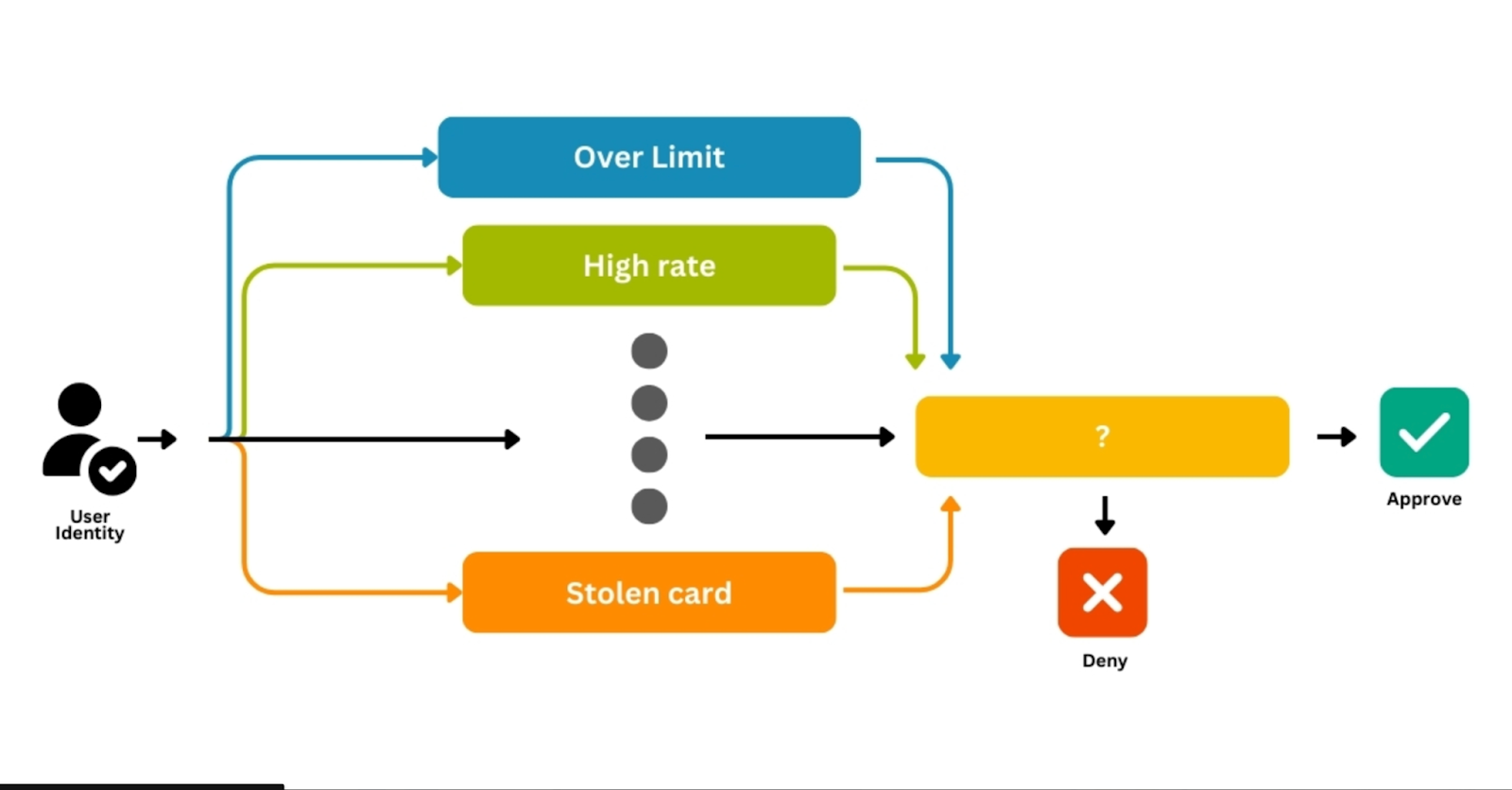
Nected represents a paradigm shift in fraud detection, introducing a rules-based methodology that simplifies the complexities associated with graph creation. By prioritizing intuitive rule structures, Nected provides a user-friendly interface for creating and managing fraud graphs, significantly reducing the learning curve.
Nected directly addresses the limitations identified within Amazon's fraud detection framework. Its approach to graph creation streamlines the process, making it more accessible and efficient. The rules-based methodology empowers you to define and modify rules easily, ensuring adaptability to evolving fraud patterns.
Implement Nected’s Rule Based Fraud Detection For Your Organization Now!
Streamlined Architecture of Nected
The architecture of Nected is designed with user convenience in mind. A user-friendly interface, coupled with intuitive tools for graph creation, distinguishes Nected. Its streamlined architecture minimizes complexities, allowing users to focus on defining rules and detecting fraud rather than grappling with intricate technicalities.
Read Also:- Which Is the Best Fraud Detection Technique In 2024?
Nected's Machine Learning Integration for Fraud Detection
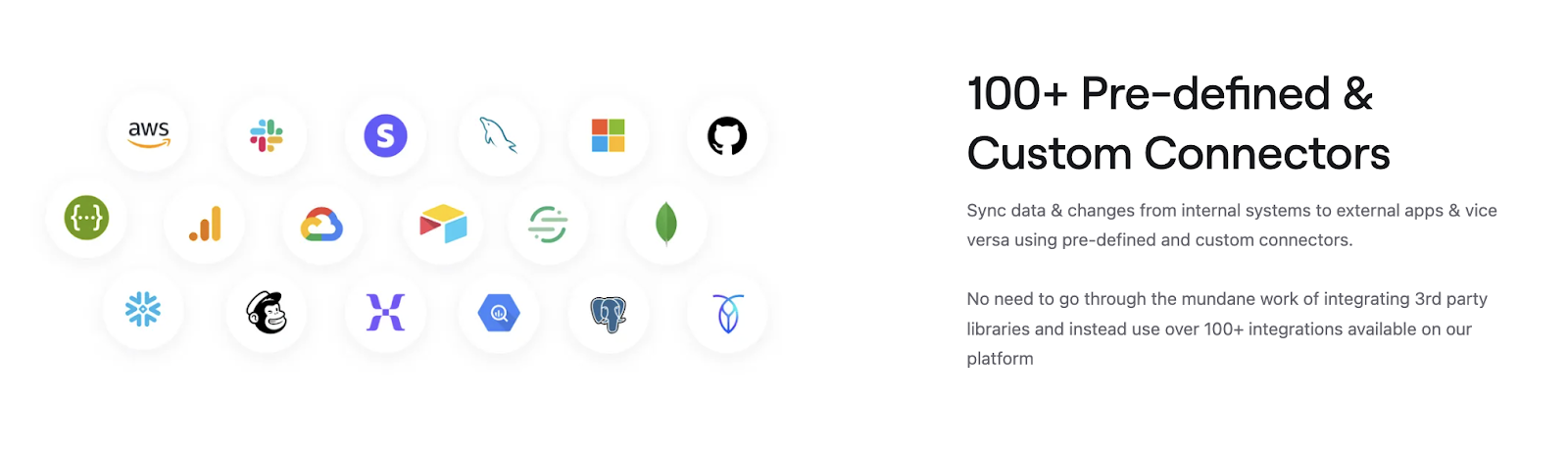
Nected goes beyond rules-based detection by integrating seamlessly with AWS Redshift for machine learning capabilities. Leveraging the power of AWS Redshift, Nected enhances its fraud detection precision. The integration enables you to apply machine learning models to identify subtle patterns and anomalies, enriching the rule-based approach with advanced analytical insights.
As you uncover the capabilities of Nected, it becomes evident that a rules-based revolution can indeed overcome the challenges posed by existing fraud detection systems, paving the way for a more accessible and efficient approach to safeguarding digital platforms against fraudulent activities.
Advantages of Nected
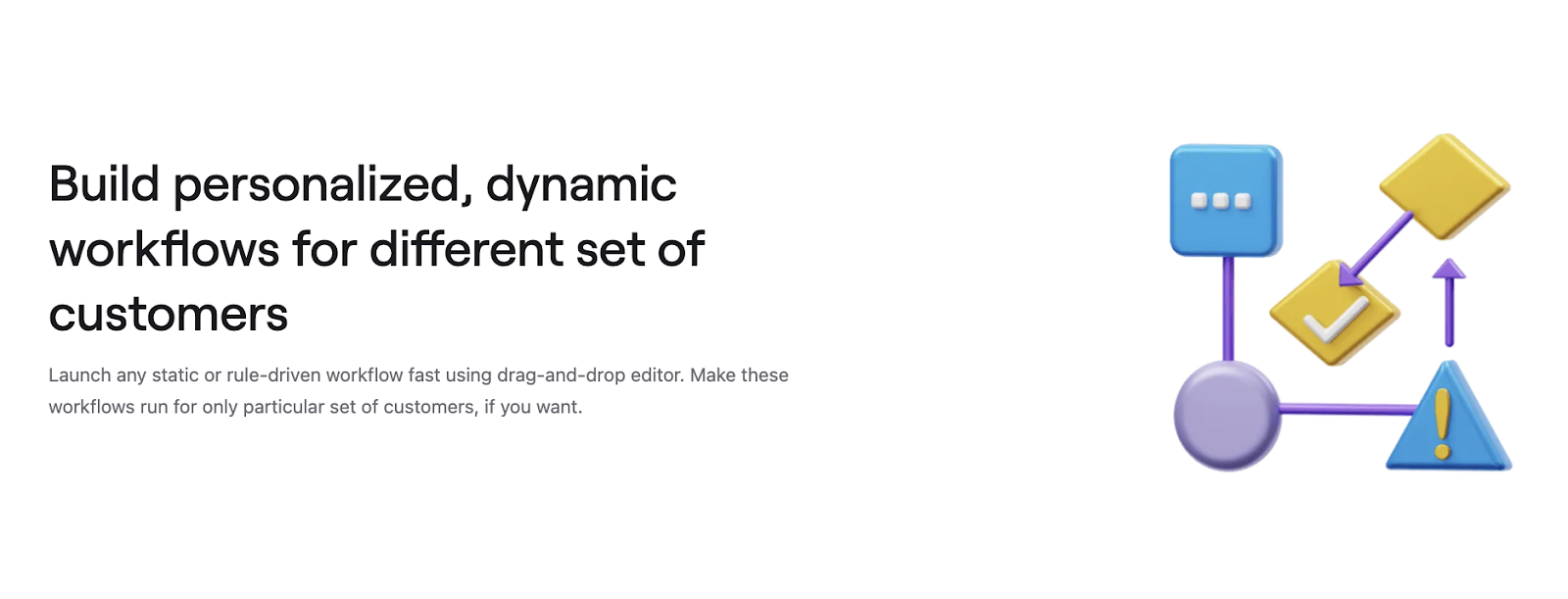
In this section, you'll explore the distinct advantages that Nected brings to the table, specifically in navigating and overcoming the limitations posed by AWS in fraud detection. Additionally, you'll know about the efficiency of Nected in real-time fraud detection scenarios.
Navigating AWS Limitations:
Nected excels in navigating and addressing the limitations inherent in AWS fraud detection systems. Its rules-based approach provides a more adaptable and user-friendly alternative, simplifying the complexities associated with graph creation and detection methodologies. Users can overcome the challenges identified in Amazon's framework, making fraud detection more efficient and effective.
The Efficiency of Real-time Fraud Detection:
One of the key strengths of Nected lies in its efficiency in real-time fraud detection. The streamlined architecture, coupled with the rules-based methodology, enables Nected to swiftly process and analyze data, making it well-suited for scenarios where immediate action is paramount. The efficiency of Nected ensures that fraudulent activities are identified and addressed promptly, minimizing potential damages.
Read Also:- Unleashing Powerful Fraud detection with Python - A Comprehensive Guide
Comparing Nected and Amazon Fraud Detection
Now, let's conduct a comparative analysis between Nected and Amazon Fraud Detection, focusing on technical aspects to provide a comprehensive understanding of their differences.
This comparative analysis provides insights into the technical nuances that differentiate Nected from Amazon Fraud Detection. Nected's emphasis on simplicity, adaptability, and real-time efficiency positions it as a compelling solution for organizations seeking an advanced and user-friendly fraud detection system.
Conclusion
In conclusion, the landscape of fraud detection is evolving rapidly, and platforms like Amazon Fraud detection and Nected are at the forefront, employing advanced technologies to safeguard digital ecosystems. While Amazon's approach to fraud detection, leveraging graph databases using Amazon Neptune and innovative services like AWS Detective, showcases remarkable strides, it's crucial to acknowledge the challenges and limitations that come with such complexity.
Nected, with its rules-based revolution, provides a refreshing perspective. Nected not only addresses the complexities faced by Amazon's systems, such as graph creation intricacies and architectural limitations but also enhances the efficiency of real-time fraud detection. Integrating seamlessly with AWS Redshift for machine learning capabilities, Nected emerges as a user-friendly and adaptable solution, simplifying the intricacies associated with fraud detection methodologies. Also Nected provides best dedicated support to its customers. If you have any doubts or issues in building rules in Nected, reach out to us at assist@nected.ai.
As organizations navigate the dynamic landscape of fraud prevention, the choice between Amazon's robust framework and Nected's rules-based approach becomes pivotal. The comparative analysis sheds light on the technical nuances, empowering decision-makers to align their fraud detection strategy with the specific needs of their digital infrastructure.
In the quest for a fraud detection solution that combines efficiency, adaptability, and user-friendliness, Nected stands as a promising contender, offering a rules-based paradigm that could revolutionize the way you combat digital fraud.

FAQs
Q1. What is AWS Detective, and how does it contribute to fraud detection on Amazon?
AWS Detective is a comprehensive service on Amazon Web Services designed to provide investigative insights into potential security issues. It plays a vital role in fraud detection by analyzing data, identifying patterns, and offering a centralized platform for security investigations. AWS Detective allows users to visualize and understand potential threats, enhancing the overall security posture of AWS environments.
Q2. Can Nected be seamlessly integrated with AWS Redshift for machine learning in fraud detection?
Yes, Nected offers seamless integration with AWS Redshift, allowing organizations to harness the power of machine learning for fraud detection. This integration enhances the precision of anomaly detection, combining rule-based methodologies with machine learning capabilities provided by AWS Redshift. The collaboration between Nected and AWS Redshift contributes to a robust and efficient fraud detection ecosystem.
Q3. How does Nected simplify graph creation complexities in fraud detection compared to Amazon's approach?
Nected simplifies graph creation by adopting a rules-based approach, reducing the inherent complexity associated with intricate relationships. Unlike Amazon's graph-based systems, which may require navigating through complex structures, Nected's intuitive methodology allows users to define rules, making the process more adaptable and user-friendly. This simplicity contributes to a more efficient and effective fraud detection system.
Q4. Which AWS service can create complex graphs for fraud detection?
Amazon Neptune is ideal for creating complex graphs for fraud detection. It’s a managed graph database service that supports detailed relationship modeling. Amazon QuickSight can also help with visualizing data in complex graphs.