Modern fraud detection techniques are indispensable to stay afloat in the present business landscape of 2024.
Why?
The PwC's Global Economic Crime and Fraud Survey 2022 revealed fraud rates to be highest in the 20 years. Consequently, businesses lose over 5% of corporate revenue to fraud each year, which amounts to US$4.7 trillion worldwide (Association of Certified Fraud Examiners), not to mention the detrimental impact on a brand's image and credibility.
That's why it's imperative for your business to adopt the latest fraud detection and prevention techniques.
In this blog, you’ll explore the main fraud analytics techniques for better fraud risk management. It will help you safeguard against various types of frauds ensuring protection for your business and assets.
Major Types of Fraud Detection Techniques Used
Financial Fraud detection techniques are many and varied. In this section, we will discuss the latest strategies that are sophisticated enough to detect and address fraudulent activities;
1. Rule-Based Fraud Detection Technique

Rules based fraud detection technique is a traditional approach to detect fraudulent activities. The best thing about a rules-based system is its simplicity and scope.
You can define the rules to monitor a wide array of scenarios and detect possible frauds. Rules-based system works on predefined criteria that you define at the beginning and when the setup detects such scenarios, it triggers an alert about a possible fraud.
Key Points on Rule-Based Fraud Detection Systems
- The rules-based system works on clear logic and interpretability. They offer greater control over fraud detection and are easy to implement.
- With rules-based systems, you can explicitly define conditions that trigger system alerts. It makes the process more transparent and open to interpretation for analysts.
- Rule-based systems like Nected are agile and can be implemented in real time to stop ongoing fraud in its tracks.
- Rule-based systems are still cost-effective compared to more resource-intensive and heavy machine-learning techniques for fraud detection.
- Rules run on exact scenarios based on defined parameters. Therefore, for preventing specific fraud, rule-based systems can be highly effective.
- Rules-based systems are highly customizable. Based on their risk tolerance and specific requirements, companies can customize rule-based systems and prevent Fraud. This adaptability makes them particularly valuable to industries with specific needs.
But you will need a robust rule-based fraud detection system and that's where Nected comes in - a cutting edge solution that is armed with powerful features and straightforward interface.
Moreover, simplicity of Nected enables businesses to get started within minutes. Its rapid implementation ability allows them to curate rules or decision tables swiftly empowering businesses to detect and avert frauds.
Implement Rule-based fraud Detection technique With Nected Now
2. Machine Learning And Artificial Intelligence

Machine Learning and Artificial intelligence represent the latest and most cutting-edge approach to fraud detection. Artificial intelligence uses machine learning models to learn about a diverse array of frauds. The system is fed with tons of historical data which it analyzes based on machine learning algorithms. Based on its previous encounters and experience, the system then analyzes the incoming stream of data for possible anomalies that may indicate fraud.
Key Points
- Machine learning techniques for fraud detection can easily recognize patterns that may indicate fraud based on historical data. They can learn about anomalies and deviations from normal patterns that could be hiding a fraud.
- AI-powered systems running and machine learning algorithms are powerful and can shift through tons of data. It gives them the ability to recognize complex patterns and subtle changes that might be indicative of unusual activities.
- AI and machine learning systems are accurate and rarely provide false positives. This makes them highly reliable in fraud detection.
- These systems are perfect for real-time system monitoring. They can process large amounts of data in real-time and make quick decisions based on the learned patterns.
- The best thing about AI and machine learning fraud detection systems is that they can be dynamic and evolve according to the changing fraud techniques and patterns. Their machine-learning capabilities allow them to adapt according to the latest data and prevent novel and more sophisticated frauds.
- One particularly effective feature of these systems is their ability to combine multiple ML and AI models. Cutting-edge techniques like gradient boosting and random forests can be applied to address a diverse array of frauds.
- These systems require minimal human intervention, making them highly efficient and effective.
- AI and Machine Learning are highly effective fraud prevention techniques in banks. Due to their ability to churn vast amounts of data, they can easily detect bank frauds like credit card scams that are often initiated through multiple accounts, locations, and devices.
3. Predictive Analytics

Predictive analytics is an effective fraud detection and prevention with data mining technique that relies on historical data to predict future frauds. It relies on powerful machine learning algorithms to learn of suspicious activities, fraudulent patterns, and deviations from normal behavior that indicate fraud. It's very effective against the evolving nature of business fraud and allows enterprises to stay one step ahead of fraudsters. It offers businesses the necessary insights they need to adopt preventive measures that could ward off incoming fraud.
Key Points
- Predictive analysis uses cutting-edge approaches like data mining, machine learning, network analysis, regression analysis, autoregressive and integrated moving average (ARIMA) models, etc. to forecast possible frauds.
- Predictive analysis is particularly beneficial in the early detection of fraud. It arms enterprises with the necessary knowledge to prevent possible fraud and minimize financial losses.
- Predictive analysis offers a broader scope for fraud detection. Systems based on predictive analysis can analyze textual data and leverage the latest approaches like entity extraction, topic modeling, and sentiment analysis to identify subtle patterns that could indicate fraud.
- These systems can even analyze geospatial data, and location-based transaction patterns to identify frauds. That's why it's highly fraud prevention techniques in banks that often involve fraudulent transactions from multiple locations.
- Data analysis techniques for fraud detection are highly scalable. They utilize machine learning models that can process humongous volumes of data in a short time. It helps them scale and adapt to the complexities of modern businesses and associated frauds.
4. Comprehensive ID Verification

Businesses are rapidly shifting over digital avenues for better growth opportunities. However, it has also paved the way for sophisticated frauds like identity thefts that can put businesses in a highly vulnerable position. Comprehensive ID verification offers a shield that enables businesses to ward off such kinds of fraud.
ID verification coupled with SCA norms is particularly suitable to fraud-proof online transaction processes by implementing multiple layers of security;
- Knowledge Factor - Something only the user knows; like password or pin.
- Possession Factor - Something the only user has; like a smart card.
- Inherence Factors - Something that the user is; like facial recognition or fingerprint authentication.
Key Points
- ID verification bolsters security efforts by mandating to implementation of at least two of the three security layers,
- Comprehensive ID verification significantly minimizes the chances of unauthorized access. It verified the user's identity via multiple authentication steps securing sensitive data.
- It minimizes the risk of credentials being stolen. Even if the login credentials are stolen, fraudsters have to deal with additional layers of authentication processes that will prevent them from accessing sensitive information.
- This technique is particularly beneficial in high-risk transaction scenarios. With ID verification in place, users have to confirm their identity before completing sensitive transactions like high-amount bank transfers. If there's a mismatch, the system raises an alert signifying a fraud.
- Id verification can be integrated with OTPs and biometric authentication systems to offer dynamic security against fraud. It ensures that only the right person with the right access and correct knowledge can pass the security layers and complete the required actions.
- The id verification process can be reinforced with Multi-Factor Authentication, Liveness detection, Secure document verification, AI and machine learning, tokens, etc. to further strengthen the system's security.
5. Behavioral Biometrics

Behavioral Biometrics is rather a new entry into the fraud detection arena. It's a contrasting approach to physical biometrics that involves human intrinsic characteristics like pupils or fingerprints. Instead, behavioral biometrics utilize human activities and deviation from normal patterns. It could be anything, keystrokes, mouse movements, screen gestures, or interactions with a particular device.
By setting baseline parameters for normal activities, behavioral biometrics systems then analyze any deviations. If it finds one it triggers an alert which could mean that someone is trying to pose as an authentic user.
Key Points
- Every person differs in their activities, hand and body movements, gestures, etc. Behavioral biometrics utilizes individualistic patterns to ensure systems safety.
- It leverages techniques like keystroke dynamics, signature analysis, gait analysis, etc. to analyze behavioral patterns and discern whether the person is authorized or not.
- Behavioral biometrics also leverage voice patterns, cursor movements, kinesthetics, etc. to ensure authorized access.
- Behavioral Biometrics systems continually analyze user interactions and gestures. Unlike static threat detection techniques, it offers continuous authentication thus providing ongoing protection against frauds.
Comparison of Fraud Detection Techniques
What Fraud Detection Methods Should You Use For Your Business?
Multiple Fraud Detection Methods are available to detect fraud in an enterprise environment. However, rule-based threat detection techniques still outshined them for its simplicity and effectiveness.
- It offers a reliable approach that lets you define what you perceive as a threat. This adaptability is still missing in other techniques like predictive analysis that run on huge datasets. Churning large data introduces complexities and uncertainties that could trigger a false alarm.
- Furthermore, rule-based fraud detection systems are more customizable and offer better control over the process. Businesses can easily tweak the system to quickly adapt to changing fraud patterns. In other systems, you don't have this flexibility, unless you command significant expertise in that particular system.
- One standout benefit of rule-based fraud detection technique is quick implementation - you can mold a rule-based fraud detection system to quickly adapt to an ongoing fraud and cut it off in its tracks before it could cause any significant damage.
- Other systems typically involve longer implementation processes. For example, an AI and machine learning system will require significant training and parameters adjusting before it can accurately detect frauds - all this will take time.
- Lastly, rules-based fraud detection systems are highly resource efficient. Other fraud detection methods like Predictive Analytics and Behavioral biometrics require extensive computational power and comprehensive hardware resources. In contrast, rules-based systems are lightweight, requiring significantly less computing power and data.
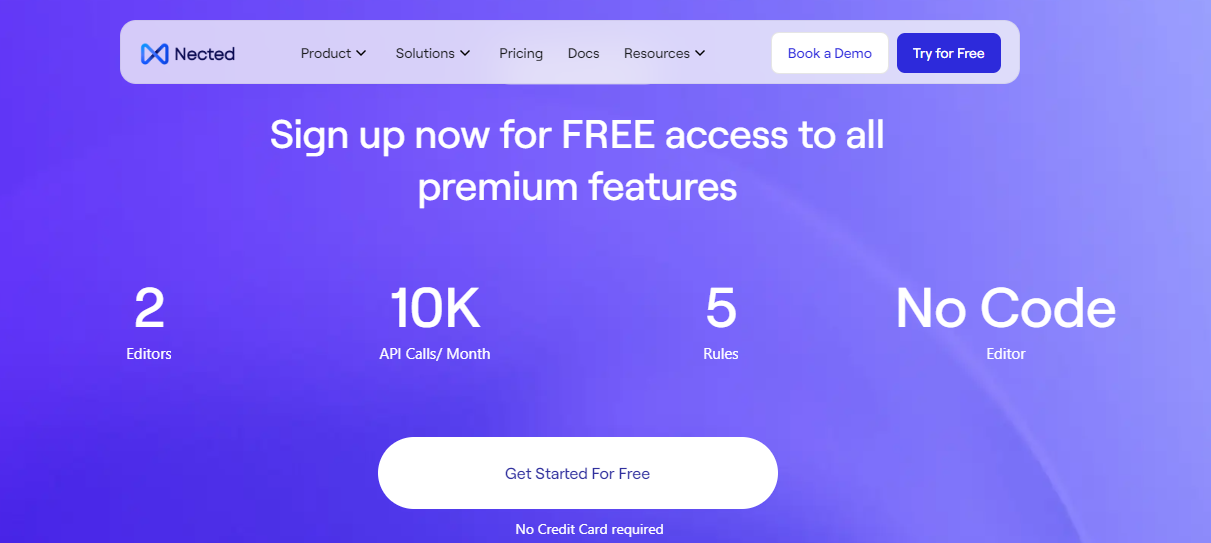
Nected offers a powerful rule-based fraud detection solution that can be tailored to curate a robust system that is accurate, reliable, and scalable. Sign up to know more.
Conclusion
Business frauds are rampant and are only going to increase with each passing day. According to the 2023 Fraud and Financial Crime Report, 69% of risk professionals and global executives expect a rapid rise in fraud and financial crime in the next 12 months.
Therefore, it's crucial to implement robust fraud detection techniques that could safeguard your business from deceptive practices.
Cutting-edge models like AI, machine learning, predictive analysis, and behavioral biometrics stand at the forefront of fraud detection. However, rule-based systems still stand out for their extreme agility and cost-effectiveness.
Nected in particular stands out as a leading rules-based fraud detection system for its intuitive functionality, advanced features, and easy implementation.
Besides, the user-friendly interface, prompt support, and real-time monitoring capabilities further help you stay strong against the escalating threats landscape.
Sign up and secure your business now!
FAQs
Q1. Is fraud detection and prevention the same thing?
No, fraud detection and prevention don't mean the same thing. Fraud prevention represents all the measures and policies adopted to minimize the possibility of fraud. On the other hand, fraud detection represents the methodology and processes implemented to detect fraud as it happens.
Q2. What techniques do fraudsters use?
Fraudsters use a variety of techniques to deceive organizations and individuals. Some of the most common techniques employed by fraudulent people are;
- Identity Thefts
- Spoofing
- Social engineering
- Cars skimming
- Phishing
- Account Takeovers
Q3. How does predictive analytics help in fraud detection?
Predictive analytics uses historical data and machine learning to identify suspicious patterns and deviations from normal behavior, allowing businesses to prevent fraud before it occurs.
Q4. What is big data fraud detection?
Big data fraud detection involves using advanced analytics and machine learning algorithms to identify and prevent fraudulent activities in large datasets. By analyzing vast amounts of data, organizations can detect patterns and anomalies that indicate potential fraud
Q5. What are fraud analysis techniques and how do they relate to big data fraud detection techniques?
Fraud analysis techniques involve methods used to examine and investigate data to identify fraudulent activities. When combined with big data fraud detection techniques, these methods leverage large volumes of data to enhance the accuracy and effectiveness of fraud detection. Big data enables more comprehensive analysis, allowing for the identification of complex fraud patterns that may not be detectable with smaller datasets.