In the world of credit assessments, the integration of machine learning adds a dynamic layer to credit scoring models. Let's begin by exploring the enhancements brought about by machine learning in credit scoring models. Rather than relying solely on static parameters, machine learning enables systems to adapt and learn from extensive datasets. This adaptability enhances the accuracy and efficiency of credit assessments, aligning them with the dynamic nature of the financial world.
Machine learning techniques, often synonymous with artificial intelligence (AI), introduce a new paradigm in credit scoring. As we explore these advancements, you'll also get to know how Nected's innovative No Code/Low Code Rule Engine is transforming credit scoring, making it more accessible and efficient for users like you.
Nected's No Code/Low Code Rule Engine empowers users like you to seamlessly build and customize credit scoring rules, eliminating traditional complexities. This user-friendly approach marks a shift in the credit scoring landscape.
Throughout this exploration, you'll discover how Nected stands out as a game-changer, bridging the gap between machine learning and credit scoring with a tool designed for you. Feel free to navigate through the sections, and let's get on a journey into the future of credit assessments.
Credit Scoring Model Using Machine Learning
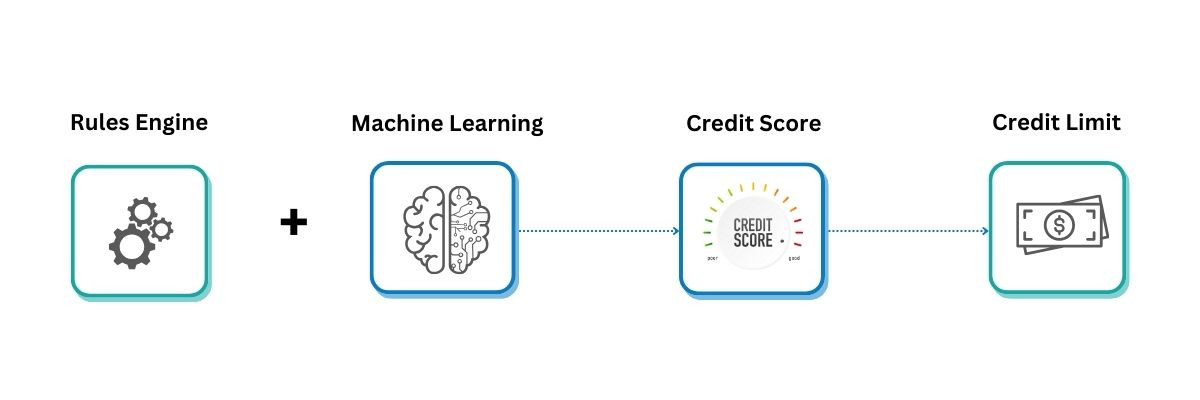
In the dynamic landscape of finance, traditional credit scoring models face limitations in capturing the complexities of borrowers' financial behavior. The emergence of machine learning has brought a large shift, introducing a more sophisticated and accurate approach to credit scoring. Let's delve into the intricacies of credit scoring methods powered by machine learning.
Machine learning introduces a new dimension by leveraging advanced algorithms to analyze vast datasets, identifying patterns and trends that may go unnoticed by conventional models. Imagine a scenario where a borrower's creditworthiness is assessed not just based on historical data but also on real-time insights derived from their financial behavior.
Consider a borrower whose financial journey exhibits non-linear patterns influenced by various factors. Traditional models may struggle to adapt to these nuances, leading to either an inaccurate assessment or an overly conservative approach. Machine learning steps in by comprehensively analyzing diverse data sources, providing a more nuanced understanding of the borrower's credit risk.
Adaptive Risk Management
In a fast-paced financial environment, adaptability is crucial. An AI based credit scoring model continuously learns from new data, adapting to changes in borrowers' financial behavior and economic landscapes. This adaptability ensures that risk management remains proactive and aligned with the ever-evolving dynamics of the financial market.
Empowering Lenders
The implementation of machine learning in credit scoring is a game-changer for lenders. It's not just about accuracy; it's about empowering financial institutions with the tools to make data-driven decisions. The synergy between machine learning and credit scoring transforms risk assessment into a strategic advantage, facilitating more precise lending decisions and ultimately contributing to a healthier financial ecosystem.
In essence, the integration of machine learning into credit scoring models signifies a shift toward a more robust and adaptive approach in the assessment of creditworthiness. As financial landscapes continue to evolve, using machine learning becomes imperative for lenders seeking a competitive edge in risk management and decision-making.
Types of Credit Scoring Models Enhanced by Machine Learning
The landscape of credit scoring has undergone a remarkable transformation with the infusion of machine learning, introducing a spectrum of models that redefine how we assess creditworthiness. Let's embark on a journey through the diverse types of credit scoring models enhanced by machine learning.
Overview of ML-Enhanced Models
Machine learning has spurred the creation of various credit scoring models, each designed to address specific challenges and nuances in assessing credit risk. Unlike traditional models that rely on fixed rules, ML-enhanced models leverage advanced algorithms to adapt and learn from evolving data, providing a more dynamic and accurate evaluation.
A critical aspect of understanding credit scoring model machine learning is to compare them with their traditional counterparts. Traditional models often rely on historical data and predefined rules, which may struggle to capture the complexities of modern financial behavior. In contrast, ML-based models excel in handling large datasets, identifying intricate patterns, and offering a predictive analysis that goes beyond historical trends.
Key Features of AI based Credit Scoring Models
AI-integrated credit scoring models introduce a host of innovative features and components that contribute to their efficacy.
Predictive Capabilities
Machine learning excels in predictive analytics, anticipating potential credit risks and opportunities. Envision a scenario where the model not only assesses current financial status but also predicts future behavior based on evolving economic conditions. This forward-looking approach enhances risk management strategies, allowing lenders to make more informed decisions.
Adaptive Learning
AI based credit scoring models exhibit adaptive learning capabilities, continuously updating their understanding of borrower behavior as new data emerges. This adaptability ensures that the model stays relevant in dynamic financial environments.
Multi-source Data Integration
Unlike traditional models that may rely on limited data sources, AI based credit scoring models can assimilate data from diverse sources, including non-traditional data points. This broader data spectrum enhances the model's accuracy in assessing credit risk.
In conclusion, the types of credit scoring models enhanced by machine learning represent a spectrum of innovative approaches to risk assessment. The comparative analysis underscores the superiority of ML-based models in adapting to contemporary financial dynamics, paving the way for a more accurate and forward-looking credit scoring landscape.
Here’s How AI Revolutionizes the Credit Scoring Industry

In the realm of credit scoring, artificial intelligence (AI) acts as a backbone, catalyzing a profound transformation in how we evaluate and mitigate credit risk. This section delves into the ways AI is revolutionizing the credit scoring industry, reshaping risk assessment methodologies and opening new frontiers for lenders.
Enhanced Risk Identification
AI based credit scoring models excel in identifying nuanced patterns and hidden correlations within vast datasets. They can discern subtle indicators of credit risk that may be overlooked by traditional models. By analyzing a myriad of factors, including non-traditional data sources, AI enhances the accuracy of risk identification. This heightened sensitivity allows lenders to proactively manage and mitigate potential credit risks, reducing instances of misclassification.
Personalized Credit Scoring
AI introduces a paradigm shift in credit scoring by enabling personalized assessments. Traditional models often apply broad criteria to evaluate creditworthiness, leading to generic risk categorizations. In contrast, AI based credit scoring models can tailor their assessments based on an individual's unique financial profile. This personalization not only improves the precision of credit scoring but also ensures a fairer and more inclusive approach to assessing credit risk.
Continuous Learning and Adaptability
One of the pros of AI based credit scoring is its ability to continuously learn and adapt. Traditional models may become outdated as financial landscapes evolve, but AI-driven models evolve in tandem with changing dynamics. They analyze new data as it emerges, adjusting their algorithms to reflect the latest market trends and borrower behaviors. This adaptability ensures that credit scoring remains robust and relevant in the face of dynamic economic conditions.
Fraud Detection and Prevention
AI plays a pivotal role in enhancing fraud detection and prevention within the credit scoring ecosystem. Machine learning algorithms can analyze transactional data in real-time, identifying anomalous patterns indicative of fraudulent activities. This proactive approach not only safeguards lenders from potential losses but also bolsters the overall integrity of the credit scoring process.
Implementing Machine Learning in Credit Scoring with Nected

In the dynamic realm of credit scoring, Nected's Credit Decision Engine not only revolutionizes the integration of machine learning (ML) but extends its capabilities to encompass robust fraud detection and prevention measures. Let's delve into a brief exploration of how Nected seamlessly combines machine learning and fraud prevention within the credit scoring landscape.
Scenario:
Imagine a financial institution aiming to bolster its credit scoring model with advanced machine learning algorithms while fortifying its defenses against potential fraud. Nected, with its No Code/Low Code Rule Engine, provides an integrated solution that addresses both these critical aspects without the need for intricate coding.
Step-by-Step Guide
Accessing Nected's Rule Engine:

Begin the journey by navigating through Nected's user-friendly interface, featuring the No Code/Low Code Rule Engine. The simplicity of the platform ensures accessibility for users with varying levels of technical expertise.
Defining Credit Scoring Rules:

Leverage Nected's rule-building capabilities to define tailored credit scoring rules and concurrently establish fraud prevention measures. The intuitive interface allows users to create rules without delving into complex coding, promoting adaptability to specific organizational needs.
Integrating Machine Learning Models

Seamlessly integrate machine learning models into the credit scoring and fraud prevention framework. Nected's flexibility allows for the incorporation of advanced algorithms, enhancing the precision of credit assessments while detecting and preventing fraudulent activities.
Nected's compatibility with connectors like RedShift enhances its capabilities. RedShift, a powerful data warehouse service, seamlessly integrates with Nected, facilitating the flow of data for both machine learning insights and fraud detection processes.
In summary, Nected offers a holistic approach to credit scoring by seamlessly integrating machine learning for enhanced risk assessments and concurrently fortifying fraud prevention measures. The platform's user-friendly features, including the scheduler and RedShift integration, empower financial institutions to navigate the complex landscape of credit scoring with ease and precision.
Conclusion
In summary, the integration of machine learning into credit scoring models is a pivotal advancement in the financial sector. Throughout this exploration, we've delved into the nuances of this integration, and we found Nected as a key player in this transformative journey.
Machine learning provides unparalleled accuracy and addresses fraud complexities, as showcased in the comparative analysis of traditional and ML-based models. The diverse credit scoring models presented offer organizations adaptable approaches to refine credit assessments.
Nected, with its No Code/Low Code Rule Engine, simplifies machine learning integration without complex coding. The user-friendly interface, coupled with features like the scheduler and RedShift connectors, positions Nected as a comprehensive solution for precise credit scoring and robust fraud prevention.In essence, Nected emerges as a strategic ally, enabling organizations to confidently embrace the future of credit scoring with innovation.
In conclusion, AI is ushering in a new age for the credit scoring industry, offering unprecedented capabilities in risk assessment, personalization, and fraud prevention. As lenders increasingly embrace AI based credit scoring models, the credit scoring landscape is poised for a transformative journey toward greater accuracy, efficiency, and inclusivity.
Optimize Credit Scoring For Your Business With Nected. Signup today!
FAQs
Q1. How does machine learning contribute to credit scoring models?
Machine learning enhances credit scoring by leveraging complex algorithms to analyze vast datasets, providing more accurate risk assessments. This results in a refined understanding of an individual's creditworthiness.
Q2. How does Nected address fraud detection in credit scoring?
Nected employs a multifaceted approach to fraud prevention. Its scheduler ensures timely rule execution, and connectors like RedShift enable the integration of machine learning for robust fraud detection, offering a comprehensive solution.