In the fast-paced world of business, keeping our operations safe from fraud is a big deal. Fraud detection is like the superhero shield that protects us from deceptive tricks, making sure everything runs smoothly. One powerful system in this fight is rules-based fraud detection – a smart way of using predefined rules to catch those sneaky fraudsters.
What's rules-based fraud detection? It's like having a set of watchful eyes that follow specific rules to spot anything fishy. If something looks off, these rules trigger an alert, letting businesses act quickly to stop potential fraud. Now, imagine having a tool that not only understands these rules but also lets you customize them as per your business needs. That's where Nected steps in – our superhero solution for rules-based fraud detection.
So, as we take this journey into the world of fraud detection, we'll see how rules-based detection is a game-changer. And with Nected by our side, it's not just about keeping things safe; it's about staying one step ahead in the battle against fraud. Let's explore the practical side of rules-based fraud detection with Nected – because securing your business should be both effective and easy.
What is Fraud Detection Rules?
Fraud detection rules are predefined sets of logical conditions used to identify potentially fraudulent activities within a system. These rules are typically based on historical data, domain expertise, and known patterns of fraudulent behavior. Fraud detection rules can include checks on transaction amounts, geographic locations, device details, user behavior, and more.
For example:
- Flagging a transaction over $10,000 from an unverified account.
- Blocking login attempts from multiple IPs in different countries within a short timeframe.
Rules-based fraud detection systems provide a structured way to monitor and mitigate risks, often acting as a first line of defense in fraud prevention strategies.
Benefits:
- Simplicity: Easy to define and implement.
- Transparency: Provides clear reasoning for flagged activities.
- Flexibility: Can be updated as fraud patterns evolve.
However, static rules can become ineffective against sophisticated or adaptive fraud tactics, necessitating dynamic systems or supplemental machine learning models.
Types of Fraud Detection
Fraud detection encompasses a variety of techniques and methodologies designed to identify and prevent fraudulent activities across different sectors. Here are the main types of fraud detection techniques:

1. Rule-Based Fraud Detection: This traditional approach uses predefined rules to identify potentially fraudulent activities. Rules can be set based on historical data and common fraud patterns. When incoming data matches these rules, the system triggers alerts for further investigation. This method is straightforward but can be limited by the scope of the rules defined.
2. Machine Learning and AI-Based Detection: Leveraging artificial intelligence and machine learning, these systems analyze large datasets to detect anomalies that may indicate fraud. They learn from historical data, allowing them to recognize complex patterns and adapt to new fraud tactics over time. This method is particularly effective for real-time monitoring and minimizes false positives.
3. Predictive Analytics: This technique utilizes historical data to forecast future fraudulent activities. It employs statistical methods and machine learning algorithms to identify suspicious patterns. By analyzing trends and behaviors, predictive analytics helps organizations anticipate potential fraud before it occurs, enabling proactive measures.
4. Anomaly Detection: Anomaly detection focuses on identifying outliers in data that deviate from established norms. It assumes that fraudulent activities often manifest as unusual patterns. Systems continuously monitor transactions or user behaviors against a baseline of normal activity, flagging any significant deviations for review.
5. Behavioral Biometrics: Unlike traditional biometrics that rely on physical traits, behavioral biometrics analyze user interactions, such as keystroke dynamics or mouse movements. By establishing a baseline of normal behavior, any significant deviations can trigger alerts, indicating possible fraudulent activity.
6. Transaction Monitoring: This technique involves real-time analysis of financial transactions to detect suspicious behavior. Automated tools assess transaction data for anomalies based on various parameters like transaction size, frequency, and geographical location.
7. Statistical Data Analysis: This method involves auditing historical data using statistical techniques to uncover fraud patterns after they have occurred. Techniques such as regression analysis and data mining help identify correlations that might indicate fraudulent activities.
8. Biometric Verification: Biometric methods such as facial recognition or fingerprint scanning provide an additional layer of security in fraud detection systems. These technologies verify the identity of users during transactions, making it harder for fraudsters to impersonate legitimate users.
Each of these fraud detection techniques has its strengths and weaknesses, often requiring a combination of methods for optimal effectiveness. Organizations typically implement multiple strategies to create a robust defense against various types of fraud, ensuring they can adapt to evolving threats in the digital landscape.
Create fraud alerts within minutes with Nected. Signup Now!
Rule-Based Systems for Fraud Detection
Rule-based fraud detection systems leverage a set of predefined business rules to identify and prevent fraudulent actions. These systems are particularly useful in scenarios where fraud patterns are well-understood and predictable.
How Rule-Based Systems Work?
Rule-based systems for fraud detection operate by using predefined conditions, or "rules," to evaluate data and identify potentially fraudulent activities. These systems are particularly effective in scenarios where fraud patterns are predictable and well-defined.

1. Input Evaluation
The system gathers data from various sources, such as user transactions, account activities, and device information. This data serves as the input for rule evaluation.
2. Rule Matching
Each data point is checked against the predefined rules. For example, a rule might specify:
If the transaction amount exceeds $5,000 and the IP address is flagged as suspicious, then trigger an alert.
The rules can include logical operators (e.g., AND, OR, NOT) and conditions based on thresholds, patterns, or anomalies.
3. Flagging or Blocking
If the system finds a match with one or more rules, it performs an action such as:
- Flagging the activity for manual review.
- Automatically blocking the transaction or login attempt.
- Sending an alert to the relevant team or system.
For instance, in e-commerce, a rule could block a purchase if the billing address doesn’t match the shipping address and the transaction originates from a high-risk country.
4. Iterative Refinement
To maintain accuracy, rule sets are periodically reviewed and updated based on new fraud patterns or feedback from false positives and negatives. This ensures that the system remains relevant and effective.
By using these steps, rule-based systems provide a transparent and structured approach to identifying and preventing fraudulent activities. However, their reliance on static rules means they are best supplemented with dynamic systems like machine learning for more adaptive fraud detection.
Advantages
- Immediate Results: Rules operate in real-time, reducing the time to detect and act on fraud.
- Control: Businesses have direct control over the rules they implement.
Limitations
- False Positives: Overly strict rules can flag legitimate actions.
- Scalability: Managing a large number of rules can become complex.
Businesses often supplement rule-based systems with machine learning models or decision engines like Nected to address these challenges to create adaptive workflows.
How to Choose a Rule-Based Fraud Detection Solution?
Selecting an appropriate rule-based fraud detection solution involves several considerations:
- Customization: Ensure the system allows for tailoring rules to your organization's unique risk factors and operational needs.
- Scalability: The solution should handle increasing transaction volumes without compromising performance.
- Integration: Compatibility with existing systems and workflows is crucial for seamless operation.
- Real-Time Processing: The ability to analyze and act on transactions instantaneously is vital for preventing fraud.
- Analytics and Reporting: Comprehensive reporting tools aid in understanding fraud patterns and refining detection strategies.
- Regulatory Compliance: To ensure adherence, the system must align with relevant legal and regulatory standards.
By carefully evaluating these factors, organizations can implement a rule-based fraud detection system that effectively safeguards against fraudulent activities while supporting business objectives.
In-House Team vs. Nected for Fraud Detection
Here’s a comparison table showcasing why Nected is a superior choice over building an in-house fraud management rules system:
So, Why Choose Nected?
- Speed and Efficiency: Nected drastically reduces implementation time, allowing you to detect fraud in real-time without waiting for months of development.
- Cost-Effective: Avoid the high costs associated with hiring and maintaining an in-house team.
- Ease of Use: With its low-code interface, Nected is accessible to both technical and non-technical users.
- Future-Proof: Nected’s dynamic rule engine and scalability make it ready to handle evolving fraud patterns effortlessly.
Investing in Nected provides immediate, scalable, and cost-efficient fraud detection capabilities, outperforming traditional in-house development approaches.
How to implement Rule-based Fraud Detection with Netced?
Let's take a scenario of Unauthorized Account Access on a website.
Open your web browser and navigate to the Nected platform. Log in with your credentials.
Step 1: Creating a New Rule

In the dashboard, locate and click on the "Rules" section.
Choose the option to "Create New Rule."
Title your rule as "Unauthorized Account Access Detection." Add a description.
Step 2: Defining Conditions for Suspicious Behavior
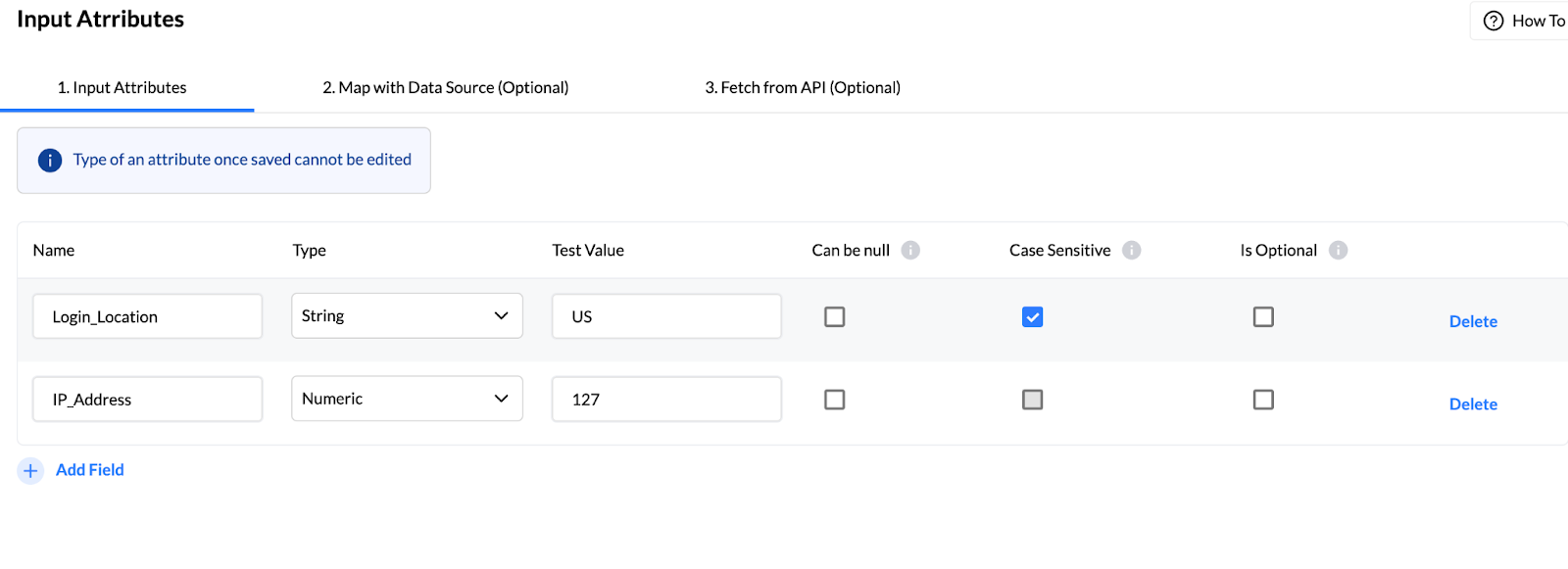
Specify conditions to identify unauthorized access (e.g., multiple logins from different locations).
Utilize Nected's rule creation interface to set criteria based on login history, geography, and time.
Step 3: Configuring Real-time Monitoring
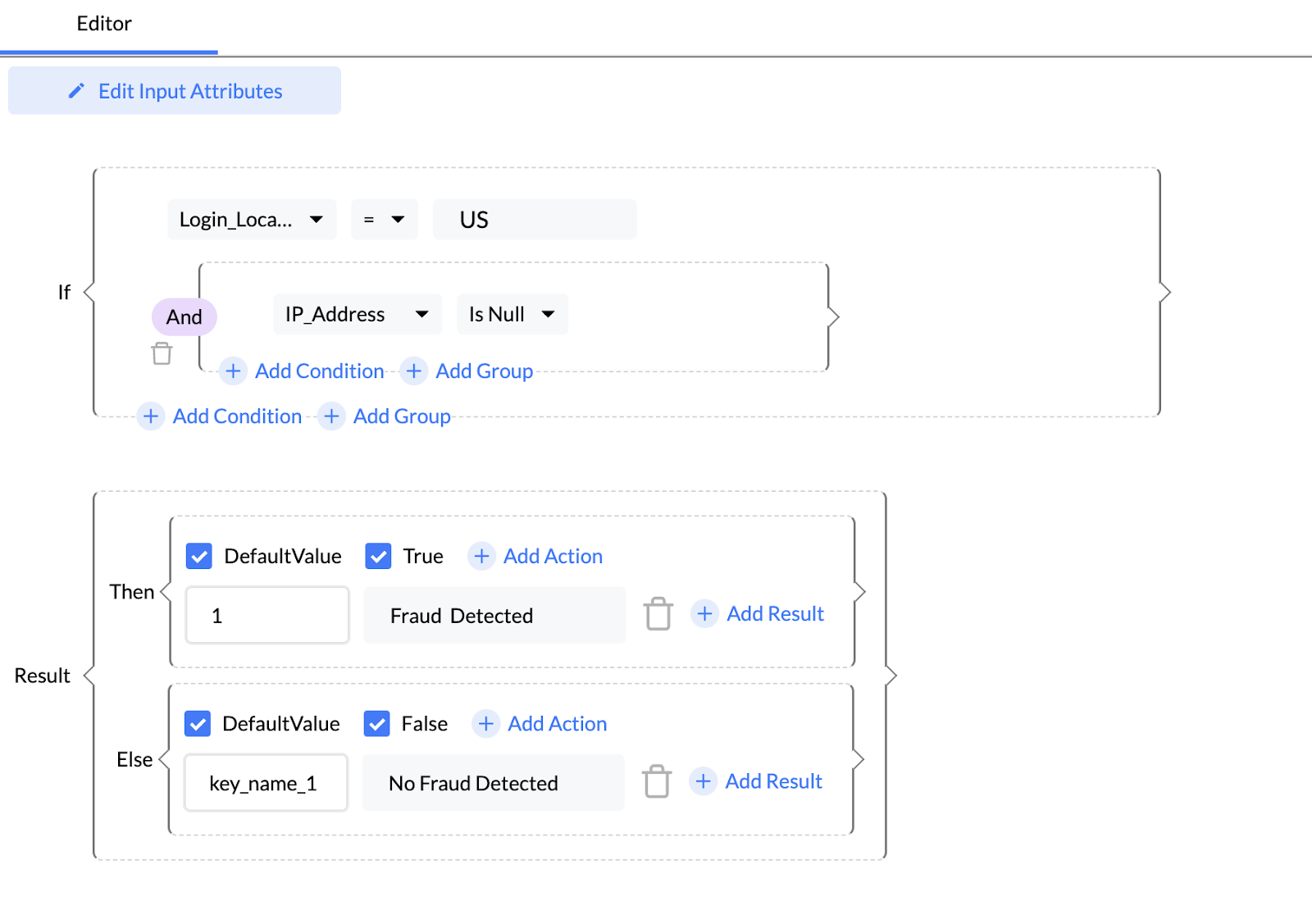
Enable real-time monitoring to ensure instant rule application.
Nected's efficient architecture ensures swift execution without system delays.
Step 4: Setting Up Alert Mechanism
Implement an alert system to notify administrators when the rule is triggered.
Configure alerts through email or preferred communication channels.
Step 5: Automated Response Setup
Establish an automated response plan (e.g., temporary account lock or additional verification).
Leverage Nected's automation capabilities for seamless execution.
Step 6: Testing in a Controlled Environment
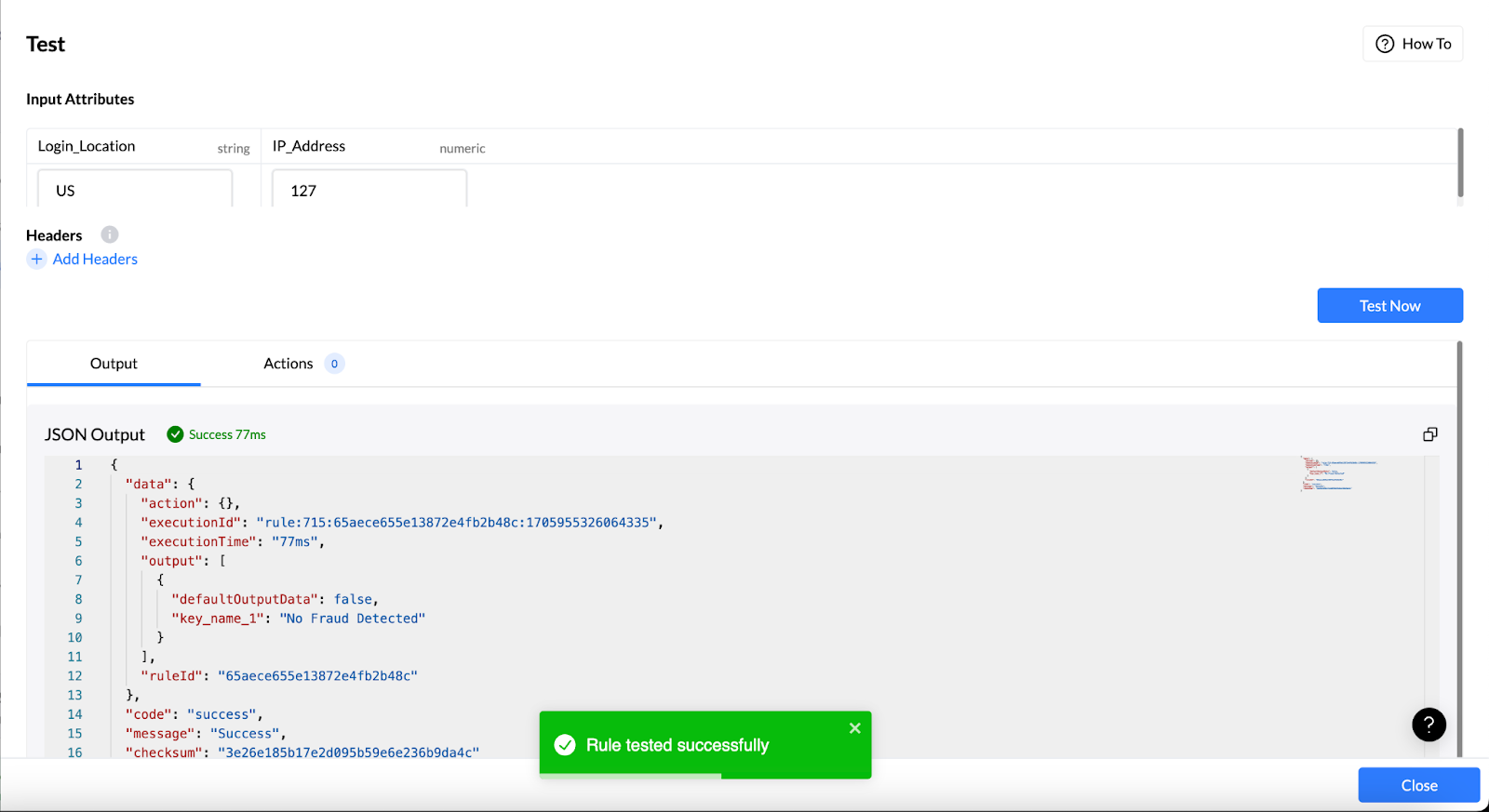
Use Nected's dedicated testing environment to simulate scenarios and ensure the rule's effectiveness.
Validate the rule without impacting real user accounts.
Step 7: Deployment and Continuous Monitoring
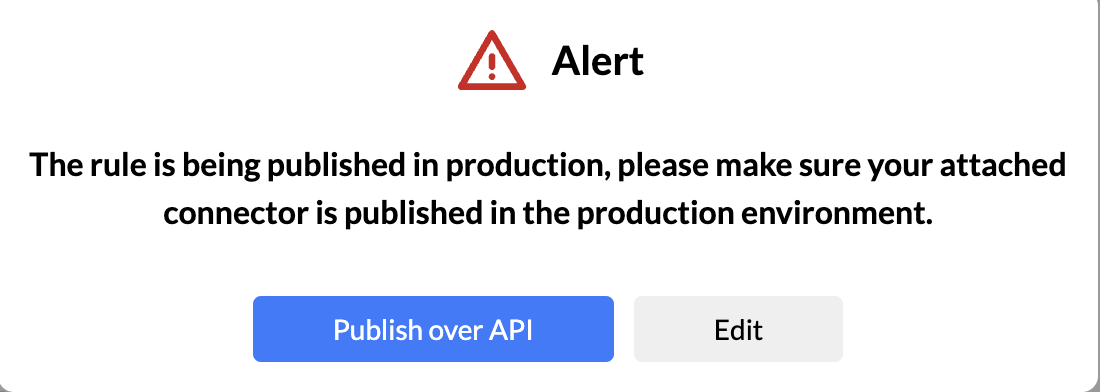
Once satisfied with testing, deploy the rule to the live production environment.
Monitor the rule continuously, making adjustments based on real-time insights.
Step 8: Regular Review and Update

Periodically review and update the rule to adapt to evolving fraud patterns.
Nected's user-friendly interface allows easy modifications for ongoing effectiveness.
This step-by-step guide provides a comprehensive overview of implementing rules-based fraud detection using Nected. From rule creation to deployment and continuous monitoring, Nected streamlines the process for enhanced security measures in organizations.
Nected's rules-based fraud detection system can be useful in various sectors. In finance, it ensures secure transactions and monitors account access. E-commerce platforms benefit from the identification of fraudulent orders and prevention of payment fraud. Healthcare can leverage Nected for safeguarding patient data and detecting fraudulent insurance claims. Telecommunications can rely on it to monitor network activities and combat SIM card fraud. Government agencies securing sensitive data, online gaming platforms detecting suspicious activities, supply chain optimization, and educational institutions ensuring integrity—all these sectors can make use of Nected's adaptable solutions, making it a practical tool in the fight against fraud.
Conclusion
In wrapping up our exploration into the domain of rules-based fraud detection, it becomes evident that safeguarding businesses against fraudulent activities is not merely a necessity but a strategic imperative. The implementation of robust fraud detection mechanisms is of supreme importance, and the choice between in-house development and leveraging cutting-edge solutions like Nected plays a pivotal role.
We've journeyed through the significance of fraud detection, the various types of fraud, and the merits of a rules-based approach. The rules-based fraud detection landscape is dynamic, and Nected, with its advanced tools and functionalities, emerges as a beacon in this space.
Nected streamlines the complex process of fraud detection by offering a user-friendly interface for crafting rules, decision tables, and rule sets. Its real-time monitoring capabilities and detailed reporting further empower businesses to stay one step ahead of potential threats.
As we deliberate on the decision to build an in-house solution or opt for Nected, the technical advantages, quick implementation, scalability, and cost-effectiveness of Nected shine through. The choice is not merely between systems but a strategic decision impacting the resilience and future-readiness of an organization.
In conclusion, the blog has endeavored to demystify the intricate landscape of rules-based fraud detection, highlighting the role of Nected as a catalyst for efficient, scalable, and cost-effective fraud prevention. As businesses navigate the complexities of security, Nected stands as a reliable ally, ensuring that organizations can fortify themselves against fraud while maintaining operational agility and efficiency. In the dynamic world of cybersecurity, Nected serves as a beacon, illuminating the path towards a secure and resilient future.
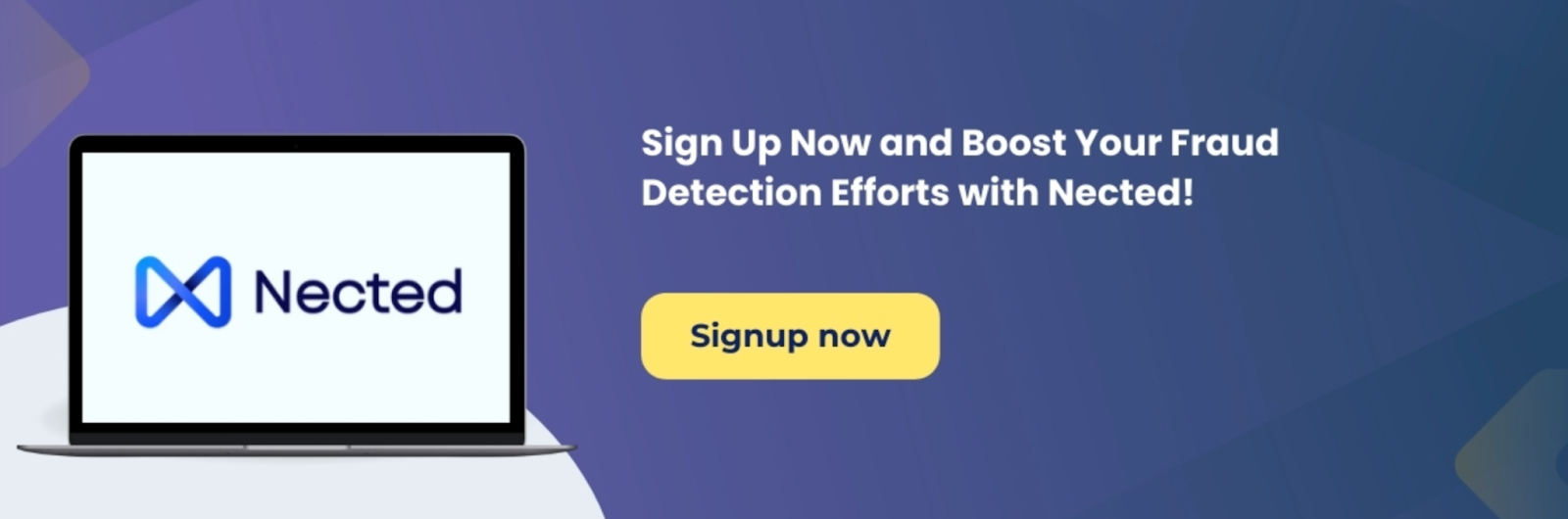
FAQs
Q1. What is a rules-based system?
A rules-based system is a type of decision-making framework that operates on predefined logic or conditions, allowing automated responses based on specified rules.
Q2. How does a rules-based fraud detection system work?
A rules-based fraud detection system relies on predefined rules and conditions to identify patterns indicative of fraudulent activities, ensuring timely detection and prevention.
Q3. Why choose Nected for rules-based fraud detection?
Nected excels in rules-based fraud detection by offering a user-friendly interface for crafting rules, decision tables, and rule sets. Its advanced tools and real-time monitoring capabilities make it a reliable choice for efficient and effective fraud prevention.
Q4. What are the limitations of rule-based fraud detection systems?
Rule-based systems may generate false positives, leading to legitimate transactions being flagged. They can also struggle to detect new fraud patterns not covered by existing rules.
Q5. How do rule-based systems differ from machine learning-based fraud detection?
Rule-based systems rely on predefined criteria, while machine learning models analyze data to identify patterns and anomalies, adapting to new fraud tactics over time.
Q6. Can rule-based and machine-learning approaches be combined?
Yes, integrating both approaches can enhance fraud detection by combining the transparency of rules with the adaptability of machine learning.
Q7. How often should fraud detection rules be updated?
Regular updates are essential to address emerging fraud tactics and changing business environments. Continuous monitoring and periodic reviews help maintain effectiveness.