A credit scoring algorithm is a systematic and rule-based procedure designed to evaluate an individual's or entity's creditworthiness. In essence, it is a set of rules that guide the assessment process, aiming to provide a numerical representation of how likely a borrower is to fulfill their financial obligations. Unlike subjective judgments, credit scoring algorithms rely on data-driven analyses and predefined criteria to ensure objectivity and consistency in evaluating credit risk.
These algorithms play a pivotal role in various financial decisions, such as lending and determining interest rates. By employing statistical models and data analysis, credit scoring algorithms streamline and enhance the credit assessment process, making it more efficient and effective. Throughout the following sections, we will delve into the technical components and intricacies that constitute a credit scoring algorithm, shedding light on the systematic approach it takes to evaluate creditworthiness.
What Credit scoring Algorithm consists of?
A credit scoring algorithm is a meticulously designed computational model aimed at objectively assessing and quantifying the creditworthiness of individuals, businesses, or entities. It involves a series of intricately connected steps, each playing a vital role in generating a comprehensive credit score. Let's delve into the detailed mechanics of each step:
- Data Collection: Collect a diverse range of financial data, encompassing traditional metrics like credit history, income, and debt, alongside non-traditional sources such as social media behavior and spending patterns. This builds an extensive dataset for a holistic financial profile.
- Weightage Factors: Assign weights to each factor based on its significance in predicting creditworthiness. For instance, factors like credit history and income may carry higher weights, reflecting their substantial impact on credit assessments.
- Scoring Model: Utilize advanced mathematical models, such as regression analysis or machine learning algorithms, to process the weighted factors. Consider interdependencies among factors for a nuanced and accurate representation of credit risk.
- Normalization: Standardize data to a common scale to ensure fair contribution from each factor, preventing variables with larger scales from disproportionately influencing the model. Normalizing data enhances fairness and objectivity in the credit scoring process.
- Score Calculation: Apply assigned weights to the normalized data systematically to calculate the final credit score. The calculated score serves as a numerical indicator of the overall credit risk associated with the individual or entity.
- Risk Categories: Categorize individuals or entities into distinct risk categories based on their credit scores. This segmentation enables lenders to make informed decisions about lending terms and conditions.
- Credit Decision: Facilitate credit decisions by determining key terms, including interest rates, credit limits, and loan terms. The credit score becomes a pivotal factor in assessing the risk and potential profitability of extending credit.
- Monitoring and Updates: Monitor changing trends in financial behavior through ongoing data analysis. Regularly update the model to ensure its ongoing relevance in a dynamic financial landscape.
In summary, a credit scoring algorithm operates as a sophisticated analytical tool, weaving together various components to deliver a nuanced and accurate assessment of creditworthiness. The subsequent section will delve into the challenges associated with implementing and maintaining such intricate algorithms.
What Factors should be considered in credit scoring algorithms?
Within the intricate framework of credit scoring algorithms, seven pivotal factors converge, collectively influencing the assessment of creditworthiness.
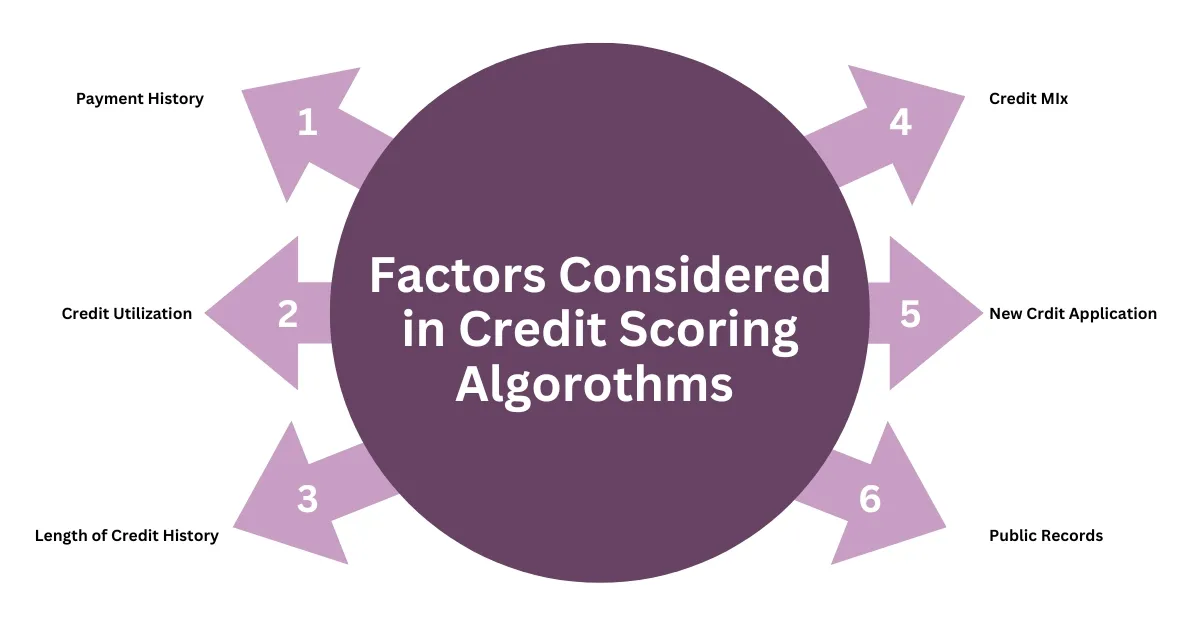
Understanding the interplay of these elements is key to unraveling the mysteries of credit scores. Let's delve into each factor, exploring real-life scenarios that vividly illustrate their impact.
- Payment History
At the forefront stands the payment history—a chronicle of financial responsibility. This factor, commanding approximately 35% of the credit score, scrutinizes the timely settlement of bills. Consistent on-time payments elevate the credit score, showcasing reliability. However, a single missed payment can cast a shadow, significantly lowering the creditworthiness.
- Credit Utilization
Credit utilization, weighing in at around 30%, mirrors the delicate balance between credit usage and limits. Maintaining a low ratio signifies responsible credit management. For instance, if Sarah utilizes a fraction of her $10,000 credit limit, her credit score benefits from the prudent handling of available credit.
- Length of Credit History
Constituting approximately 15% of the credit score, the length of credit history acts as a time capsule. A lengthier credit history provides lenders with a richer dataset for evaluating creditworthiness. Mark, with over a decade of responsible credit usage, stands in favorable contrast to a newcomer in the credit arena.
- Credit Mix
The credit mix, accounting for around 10%, advocates for a diversified portfolio of credit types—credit cards, mortgages, loans, etc. This diversity underscores an individual's ability to manage varied financial obligations.
- New Credit Applications
New credit applications, composing about 10%, introduce an inquiry quotient. Multiple applications within a short span can trigger concerns, hinting at potential financial distress.
- Public Records
Public records, encompassing bankruptcies, tax liens, and civil judgments, cast a shadow on credit scores. These negative imprints signal financial instability and unpaid obligations.
Navigating the intricacies of these factors provides individuals with the foresight to make informed financial decisions, fortifying their creditworthiness for a robust financial future.
How does FICO's Credit Scoring Algorithm evaluate your Financial Profile?

The FICO credit scoring algorithm employs a multifaceted approach, akin to a sophisticated mathematical model with various weighted components. Let us break down the intricacies to understand what factors FICO, the standard for credit scoring, uses to evaluate credit score.
- Payment History (35%): This parameter meticulously evaluates the chronology of your credit payments. Delving into granular details, it assesses the punctuality of your payments, assigning a numerical value to each instance.
- Credit Utilization (30%): This facet involves a rigorous examination of the ratio between your outstanding credit balances and the total available credit. Complex algorithms intricately compute this ratio, providing a comprehensive snapshot of your financial behavior.
- Length of Credit History (15%): The algorithm systematically computes the duration of your credit accounts, utilizing intricate algorithms to assign numerical values to the longevity of each account. Longer histories contribute positively to the overall score.
- Types of Credit Used (10%): Employing a categorization algorithm, this component scrutinizes the diversity of your credit portfolio. Each credit type is assigned a specific weight, contributing to a holistic evaluation of your credit profile.
- New Credit (10%): The algorithm employs statistical models to scrutinize the frequency of new credit applications. A higher frequency is algorithmically interpreted as a potential risk factor, influencing the overall credit score.
In essence, the FICO credit scoring algorithm operates as a finely tuned mathematical engine, where each parameter is meticulously calculated to generate a comprehensive credit score.
What Custom Credit Scoring Algorithm offers?
In the landscape of credit assessment, custom credit scoring algorithms stand out as a specialized approach designed for precision and tailored evaluation. Unlike conventional, one-size-fits-all models, custom algorithms offer a personalized analysis, taking into account specific factors that may be crucial for a particular industry or borrower profile.
- Tailored Precision: Custom credit scoring algorithms are crafted to meet the distinctive needs of lenders or businesses. By incorporating industry-specific metrics, these algorithms ensure a more accurate reflection of creditworthiness, leading to informed lending decisions.
- Niche Focus: These algorithms are often designed to cater to niche markets or specialized lending, where traditional scoring models may fall short. Whether it's evaluating the creditworthiness of small businesses, startups, or individuals with unconventional financial patterns, custom algorithms provide a more nuanced and insightful assessment.
- Incorporating Non-Traditional Data: While traditional credit scoring models rely on standard financial data, custom algorithms have the flexibility to incorporate non-traditional sources. This may include data from unconventional financial transactions, industry-specific performance indicators, or other relevant metrics that contribute to a comprehensive evaluation.
- Adaptable to Changing Dynamics: The adaptability of custom credit scoring algorithms shines when faced with evolving economic landscapes. These models can be adjusted and fine-tuned to accommodate changes in market conditions, ensuring that the credit assessment remains relevant and reliable over time.
- Enhanced Decision-Making: By tailoring the evaluation criteria, custom credit scoring algorithms empower lenders to make more informed decisions. This is particularly valuable when dealing with unique borrower profiles or industries where traditional models may not capture the full picture.
- Integration with Technology: Custom algorithms can seamlessly integrate with modern technological solutions, enhancing efficiency in the credit assessment process. This may include incorporating artificial intelligence, machine learning, or other advanced technologies to improve accuracy and speed in decision-making.
In summary, custom credit scoring algorithms offer a bespoke approach to credit assessment, providing tailored precision, niche-focused analysis, adaptability to changing dynamics, and integration with cutting-edge technology. As the financial landscape continues to evolve, the role of custom algorithms becomes increasingly pivotal in driving informed and nuanced credit decisions.
Challenges in Credit Scoring Algorithm Implementation
The implementation and maintenance of credit scoring algorithms present several challenges, impacting their effectiveness and accuracy:
Algorithmic Learning Curve:
- Challenge: The intricacies of credit scoring algorithms, including statistical methods and machine learning models, pose a steep learning curve.
- Impact: Financial institutions may face challenges in training staff to understand and effectively utilize complex algorithms, potentially leading to suboptimal implementation.
Database Size and Complexity:
- Challenge: Managing and processing extensive datasets, including both traditional and alternative data, can be resource-intensive.
- Impact: Large databases may result in slower processing times, affecting the efficiency of credit assessments and decision-making.
Normalization Challenges:
- Challenge: Ensuring fair and accurate representation of diverse data sources during normalization is a critical aspect of algorithmic implementation.
- Impact: Inaccurate normalization can skew the weighting of factors, potentially leading to biased credit scores and compromised assessment outcomes.
Navigating these challenges demands a strategic approach to ensure the seamless functioning of credit scoring algorithms. The focus is on overcoming hurdles in credit scoring algorithm implementation for practical application in real-world scenarios.
5 Step Credit Scoring Algorithm with Nected
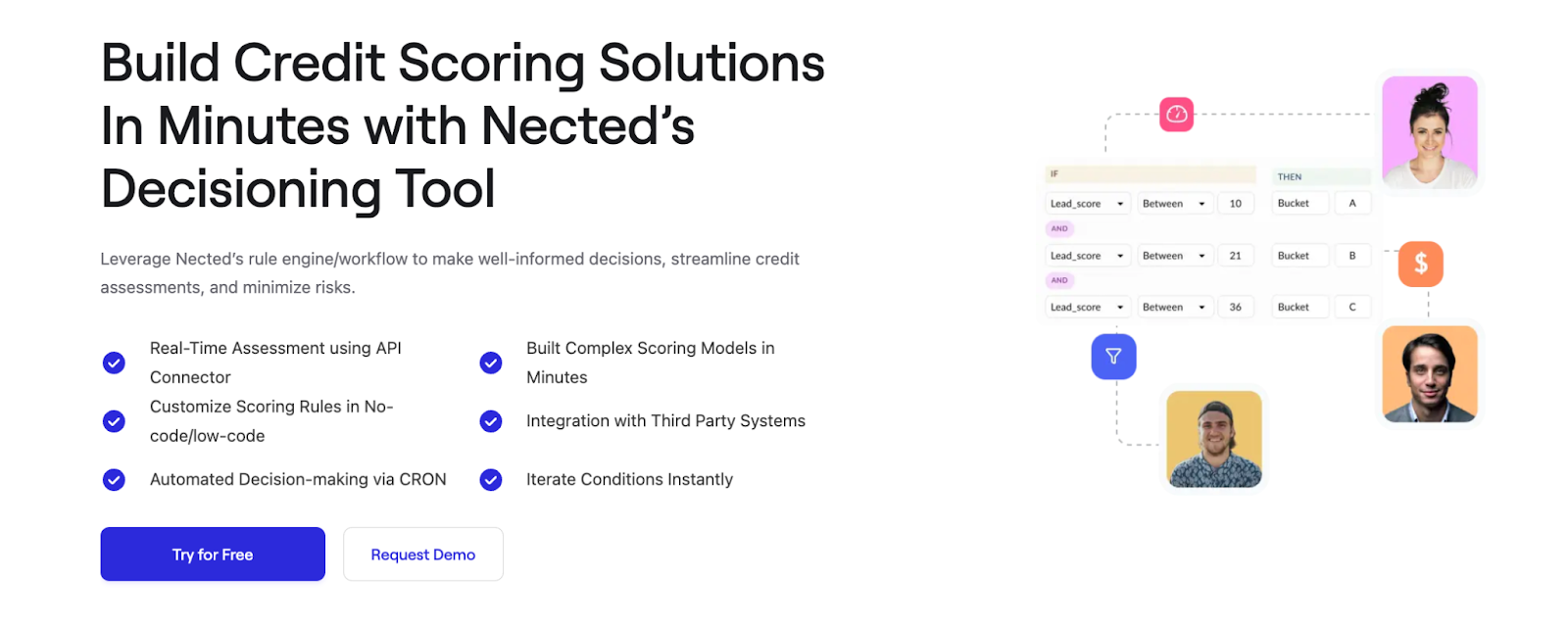
Nected streamlines the credit scoring process by offering a comprehensive platform with user-friendly features. Here's a brief overview of how Nected eases the credit scoring journey:
- Connect Integrations: Seamlessly integrate Nected with databases like MongoDB, Postgres, Redshift, and MySQL and many more using connectors. Additionally, you can create connectors for REST APIs, enabling integration with systems supporting HTTP calls. The platform provides both staging and production environments for convenient changes.
- Unify Data via Datasets: Utilize custom database queries within Nected to retrieve and unify data from your databases via Datasets. This process facilitates binding data against rules, eliminating the need to pass data points separately.
- Rules Creation: Build complex rules effortlessly in a no-code editor within Nected. The platform supports simple rules, decision table rules, and rule sets through an intuitive interface. Moreover, it accommodates custom JavaScript code for defining intricate rule conditions and outputs. All rules are instantly exposed via APIs for seamless integration with existing workflows.
- Rules Actions: Based on the outcomes of the rules, Nected allows you to trigger database actions. This feature enhances the adaptability of the credit scoring model, ensuring that the decision-making process aligns with the established rules.
- Testing and Validation: Nected provides robust testing and validation capabilities, allowing users to validate rules before deploying them in live environments. This ensures accuracy and reliability in credit scoring decisions.
Nected's user-friendly approach, coupled with its powerful features, contributes to the efficiency and effectiveness of credit scoring processes. For more details, explore Nected's rules engine Documentation or watch a video on how credit scoring is done with Nected.
Conclusion
In conclusion, credit scoring algorithms play a pivotal role in evaluating the creditworthiness of individuals and businesses. The step-by-step procedures involved in data collection, weighting factors, scoring models, normalization, score calculation, risk categories, credit decisions, and continuous monitoring ensure a nuanced and accurate assessment.
The challenges in implementing credit scoring algorithms, including a steep learning curve and managing large databases, underscore the need for sophisticated solutions. However, advancements in technology, exemplified by platforms like Nected, offer substantial support in overcoming these challenges.
Nected stands out as a transformative solution that simplifies and enhances the credit scoring process. With its intuitive features, including connectors for seamless integration, unified data handling via datasets, and a versatile no-code editor for rule creation, Nected empowers businesses to streamline their credit scoring workflows.
Embrace Nected for a future-proof credit scoring system that combines sophistication with user-friendly design.
FAQs
Q1. How do credit scoring algorithms determine my creditworthiness?
Credit scoring algorithms evaluate various factors, including payment history, credit utilization, length of credit history, credit mix, new credit applications, and public records. Each factor is weighted differently to calculate a numerical representation of your creditworthiness.
Q2. Do different credit bureaus use the same scoring algorithms?
While there are common credit scoring models like FICO and VantageScore, each credit bureau may use its variations. The proprietary algorithms developed by credit bureaus may consider additional data specific to their reports.